How to Write Data Analysis in Research: Best Practices
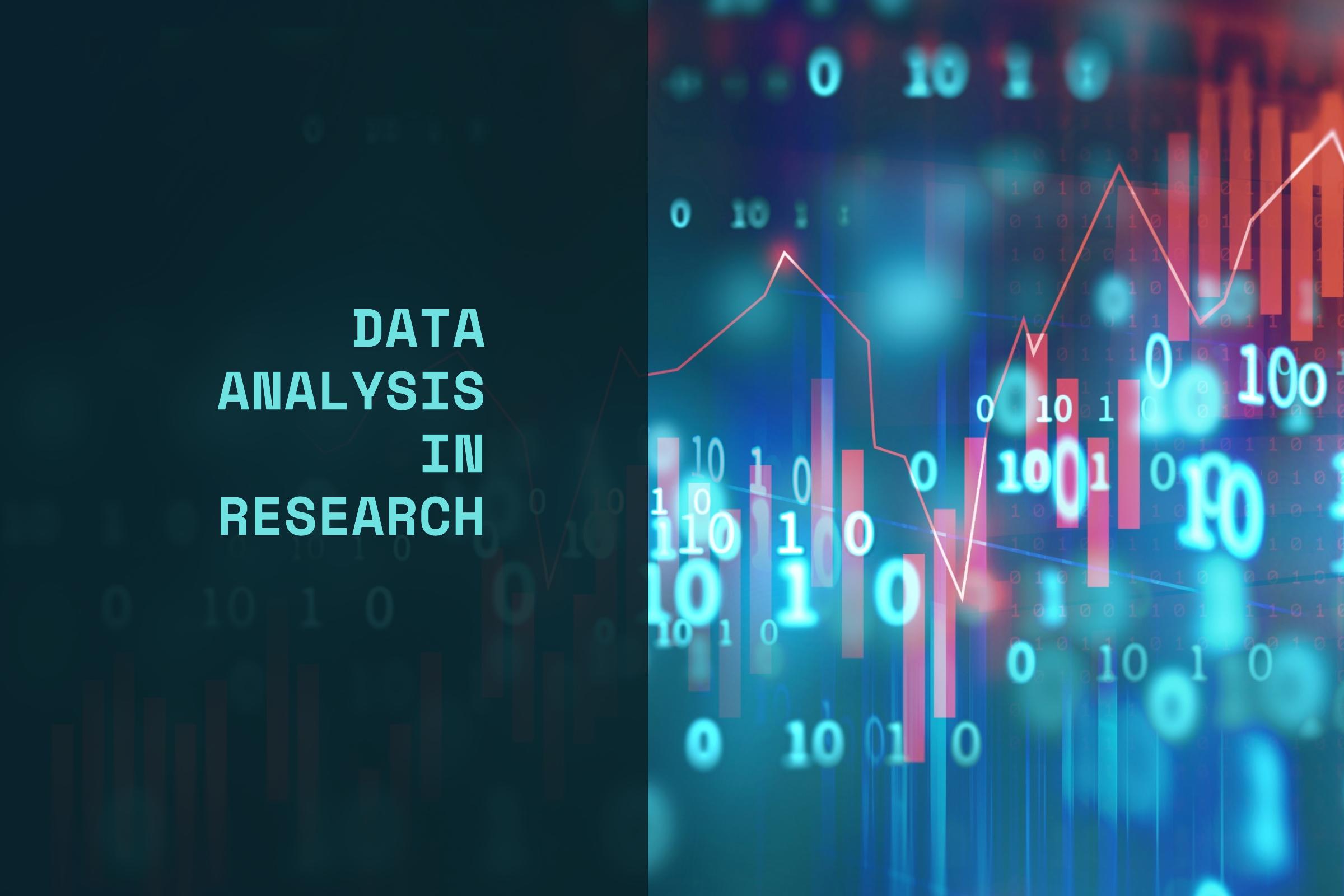
Nov. 9, 2024
Data analysis is a section with which one has to deal in every research project. This process is particularly overwhelming to novices. Learning how to write data analysis in research just might be that difference between a successful study and one that is not. By the end of this course, you will have mastered the various types of approaches of research, planned your research, and made major steps on how to carry out data analysis to get appropriate results accordingly.
The following walks you through conducting and writing-up data analysis for research. You'll learn how to plan your analysis-including how to select the right variables to study-and how to present findings. By the end of learning good practices, you can be confident to take up the challenge of data analysis and design a study that shall contribute to changing the knowledge base within your field.
Understanding the Basics of Data Analysis
What is data analysis in research?
Data analysis in research is the process of systematically examining, cleaning, transforming, and interpreting data to uncover valuable insights and draw meaningful conclusions. It's a crucial step in the research process that helps you make sense of the information you've collected. When you're learning how to write data analysis in research, it's important to understand that this process involves applying statistical techniques to describe, illustrate, and evaluate your data.The main goal of data analysis is to convert raw data into a format that's easy to understand, more legible, and supports decision-making. This process begins with a clear research question or hypothesis, guiding your approach to data collection and analysis. By scrutinizing your data, you can identify patterns, relationships, and trends that might not be immediately apparent.
Types of data analysis
There are several types of data analysis, each serving a different purpose in your research. Understanding these types can help you choose the most appropriate approach for your study:Descriptive Analysis: This type summarizes the main characteristics of your dataset. It involves calculating measures of central tendency (like mean, median, and mode) and measures of spread (such as variance and standard deviation).
Diagnostic Analysis: This approach digs deeper into your data to uncover the causes of specific outcomes or events. It helps you understand why certain patterns or anomalies occur in your data.
Predictive Analysis: This type uses historical data to make predictions about future outcomes. It employs statistical models and machine learning algorithms to identify patterns and trends in your data.
Prescriptive Analysis: Building on predictive analysis, this approach provides recommendations or optimal solutions based on predicted outcomes.
Importance of data analysis in research
Data analysis plays a vital role in various aspects of research:Informed Decision Making: By analyzing your data, you can make evidence-based decisions rather than relying on intuition or assumptions. This approach leads to more reliable and effective outcomes in your research.
Problem Solving: Data analysis helps you identify and solve problems by uncovering root causes and detecting anomalies in your research data.
Performance Evaluation: Through data analysis, you can track progress, measure success, and evaluate the performance of your research methods or interventions.
Innovation: The insights gained from data analysis can drive innovation in your research field, leading to new hypotheses, research questions, or methodologies.
Risk Mitigation: By identifying potential risk factors in your data, you can take proactive measures to minimize negative impacts on your research outcomes.
Understanding these basics of data analysis will provide a solid foundation as you learn how to write data analysis in research. Remember, the key to effective data analysis lies in choosing the right approach for your research design and variables and interpreting your results accurately within the context of your research methodology.
Planning Your Data Analysis
Defining research questions and hypotheses
To write data analysis in research effectively, you need to start by defining clear research questions and hypotheses. These elements serve as the foundation for your study and guide your data analysis steps. When developing research questions, focus on current trends and cutting-edge studies in your field. Excellent research questions are specific and concise, providing a clear basis for developing hypotheses.Your research questions should be based on a comprehensive literature search and an in-depth understanding of the problem you're investigating. Initially, you might write descriptive questions, which can later be developed into inferential questions. Remember, well-constructed hypotheses are based on previous reports and verify the research context.
Choosing appropriate data collection methods
Once you've defined your research questions and hypotheses, the next step in your data analysis in research methodology is to choose appropriate data collection methods. Your choice of method depends on various factors, including your research goal, the subjects you want to study, and the type of data you need to collect.Common data collection methods include surveys, interviews, focus groups, experiments, and secondary data analysis. Each method has its strengths and weaknesses, so consider the time you have for research and the balance between these factors. For example, surveys help you collect more data quickly, but they may lack details. On the other hand, interviews or observations provide richer information but take more time to conduct and analyze.
Selecting suitable analysis techniques
The final step in planning your data analysis is selecting suitable analysis techniques. Your choice of technique depends on your research design, the types of variables you're dealing with, and the distribution of your data. In quantitative research, you'll likely use statistical analysis to summarize your sample data, make estimates, and test hypotheses.Descriptive statistics can help you summarize your sample data in terms of distribution, central tendency, and variability. Inferential statistics allow you to make estimates about the population based on your sample data and test hypotheses about relationships between variables. Common techniques include regression and correlation tests for associations between variables, and comparison tests like t-tests and ANOVAs for differences in outcomes between groups.
For qualitative research, your analysis might involve thematic analysis or discourse analysis. Thematic analysis focuses on identifying key themes in your data, while discourse analysis puts the data in context and analyzes different levels of communication.
Remember, your choice of analysis technique should align with your research questions and the type of data you've collected. By carefully planning your data analysis approach, you'll be better equipped to write a comprehensive and insightful data analysis in your research.
Conducting the Data Analysis
When you're ready to conduct your data analysis in research, it's crucial to follow a systematic approach. This process involves several key steps that will help you extract meaningful insights from your collected data.
Preparing and cleaning the data
The first step in conducting data analysis is to prepare and clean your data. This involves examining your dataset for any errors, inconsistencies, or missing values. You'll need to address these issues to ensure the accuracy and reliability of your results. Start by checking for duplicate entries and removing them. Look for any outliers that might skew your analysis and decide whether to keep or remove them based on your research design.Next, handle any missing data. Depending on the extent of missing values, you might choose to impute them using statistical methods or remove the affected observations entirely. Be sure to document any changes you make to your dataset during this process.
Applying statistical methods
Once your data is clean and ready for analysis, it's time to apply appropriate statistical methods. The choice of statistical tests depends on your research approach and the types of variables you're working with. For quantitative data, you might use parametric tests like t-tests or ANOVA if your data meets certain assumptions, such as normal distribution and homogeneity of variance.If your data doesn't meet these assumptions, consider using non-parametric alternatices. For example, you might use a Mann-Whitney U test instead of a t-test, or a Kruskal-Wallis test instead of ANOVA. Remember that the statistical methods you choose should align with your research design and help answer your research questions.
When applying statistical methods, it's important to consider the sample size and the power of your tests. Larger sample sizes generally provide more reliable results and increase the likelihood of detecting significant effects if they exist.
Interpreting the results
The final step in conducting data analysis is interpreting your results. This involves examining the output of your statistical tests and making sense of what they mean in the context of your research. Start by looking at the test statistics and p-values to determine if your results are statistically significant.However, don't rely solely on p-values. Consider the effect size, which tells you about the magnitude of the relationship or difference you've found. This can be particularly important when working with large sample sizes, where even small effects can be statistically significant.
When interpreting your results, always refer back to your original research questions and hypotheses. Consider how your findings support or contradict your initial expectations. Look for patterns or trends in your data that might provide additional insights beyond your primary research questions.
Remember that data analysis in research methodology is an iterative process. You may need to revisit earlier steps, refine your analysis, or conduct additional tests based on your initial findings. By following these data analysis steps and maintaining a systematic approach, you'll be well-equipped to extract valuable insights from your research data.
Presenting Your Findings
Creating effective data visualizations
When presenting your data analysis results, creating effective data visualizations is crucial to communicate your findings clearly. Visualizations help convey complex information succinctly and highlight important trends or patterns in your research. To create impactful visualizations, consider your audience and the purpose of your presentation. Choose the right type of chart or graph that best represents your data and supports your research approach.Bar charts are excellent for comparing categories within a single measure, while line graphs effectively show trends over time. Maps can be useful for visualizing location-specific data, and histograms help display the distribution of continuous variables. When designing your visualizations, use color strategically to emphasize key points and guide the viewer's attention. However, avoid overusing color, as it can lead to confusion or distract from the main message.
Ensure your visualizations are self-explanatory by including clear titles, legends, and labels. Use intuitive colors and shapes that make sense to the viewer, allowing them to process the information quickly. Remember to keep your visualizations simple and focused on the most important aspects of your data analysis results.
Writing a clear and concise analysis report
When writing your data analysis report, clarity and conciseness are key to effectively communicating your findings. Structure your report logically, using headings and subheadings to guide readers through your content. Begin with an introduction that outlines your research approach and objectives, then present your findings in a clear and organized manner.Use plain language and avoid jargon to ensure your report is accessible to a wide audience. Be specific and objective in your writing, focusing on the most important insights from your data analysis. When presenting numerical data, consider using tables for precise values and graphs for highlighting trends or patterns.
To make your report more engaging, incorporate relevant examples or case studies that illustrate your findings. This helps readers understand the practical implications of your research and how it relates to real-world scenarios.
Addressing limitations and future research directions
Acknowledging the limitations of your study is an essential part of presenting your findings. Discuss any constraints or weaknesses in your research design or methodology that may have affected your results. This demonstrates your awareness of potential issues and helps readers interpret your findings more accurately.When addressing limitations, explain how they might have impacted your study and propose possible ways to overcome these challenges in future research. This shows that you've considered the broader implications of your work and are committed to advancing knowledge in your field.
Finally, suggest directions for future research based on your findings and the gaps you've identified in the current literature. This helps to contextualize your work within the broader research landscape and provides valuable insights for other researchers in your field.
By following these guidelines for presenting your findings, you'll be able to effectively communicate the results of your data analysis in research, ensuring that your audience can understand and act upon your insights.
Conclusion
Mastering data analysis in research is a journey that requires careful planning, execution, and presentation. This guide has outlined the key steps to write data analysis in research, from understanding the basics to presenting your findings effectively. By following these best practices, beginners can develop the skills needed to conduct meaningful research and make a significant contribution to their field.
The process of data analysis has a profound impact on the quality and reliability of research outcomes. It enables researchers to uncover hidden patterns, test hypotheses, and draw evidence-based conclusions. As you continue to refine your data analysis skills, remember that practice and persistence are crucial. Each research project provides an opportunity to learn and improve, ultimately leading to more insightful and impactful research in your chosen field.
Sign up for a free Article tool trial for the best plagiarism detection and other tools helpful for a unique writing.
FAQs
1. How is data analysis structured in a research study?
To effectively write data analysis for a research paper, follow these steps:
• Develop your hypothesis and research design.
• Gather data from a representative sample.
• Summarize and analyze your data.
• Use inferential statistics to test hypotheses and draw conclusions.
• Interpret and present your findings.
2. What are the steps involved in writing about data analysis techniques in research?
Writing about data analysis techniques involves several key steps:
• Define the scope of your study.
• Collect necessary data.
• Process the data for analysis.
• Conduct the analysis.
• Draw conclusions and interpret the results.